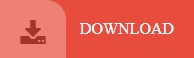
Lefort M, Sharmin S, Andersen JB, Vukusic S, Casey R, Debouverie M, Edan G, Ciron J, Ruet A, De Sèze J, Maillart E, Zephir H, Labauge P, Defer G, Lebrun-Frenay C, Moreau T, Berger E, Clavelou P, Pelletier J, Stankoff B, Gout O, Thouvenot E, Heinzlef O, Al-Khedr A, Bourre B, Casez O, Cabre P, Montcuquet A, Wahab A, Camdessanché JP, Maurousset A, Ben Nasr H, Hankiewicz K, Pottier C, Maubeuge N, Dimitri-Boulos D, Nifle C, Laplaud DA, Horakova D, Havrdova EK, Alroughani R, Izquierdo G, Eichau S, Ozakbas S, Patti F, Onofrj M, Lugaresi A, Terzi M, Grammond P, Grand'Maison F, Yamout B, Prat A, Girard M, Duquette P, Boz C, Trojano M, McCombe P, Slee M, Lechner-Scott J, Turkoglu R, Sola P, Ferraro D, Granella F, Shaygannejad V, Prevost J, Maimone D, Skibina O, Buzzard K, Van der Walt A, Karabudak R, Van Wijmeersch B, Csepany T, Spitaleri D, Vucic S, Koch-Henriksen N, Sellebjerg F, Soerensen PS, Hilt Christensen CC, Rasmussen PV, Jensen MB, Frederiksen JL, Bramow S, Mathiesen HK, Schreiber KI, Butzkueven H, Magyari M, Kalincik T, Leray E. The choice of the estimand should drive the choice of the method.Īverage treatment effect average treatment on the treated inverse probability weighting matching positivity assumption propensity score. We show that this empirical result is supported by theory.Īlthough both approaches are recommended as valid methods for causal inference, propensity score-matching for ATT and inverse probability of treatment weighting for average treatment effect yield substantially different estimates of treatment effect. However, when treatment effect varies according to the propensity score (E is not constant, Y being the outcome and g(X) the propensity score), propensity score matching ATT estimate could strongly differ from the inverse probability of treatment weighting-average treatment effect estimate. From simulations, we concluded that positivity bias was of limited magnitude and did not explain the large differences in the point estimates. Potential reasons were (1) violation of the positivity assumption (2) treatment effect was not uniform across the distribution of the propensity score. We used Monte Carlo simulations to investigate the important differences in the two estimates.Ĭontinuous positive airway pressure application increased hospital mortality overall, but no continuous positive airway pressure effect was found on the treated. We applied the two propensity score methods to assess the effect of continuous positive airway pressure on mortality in patients hospitalized for acute heart failure. We illustrate how different estimands can result in very different conclusions. *First approach to calculate ATU (ATET with subpopulation if treatmentvariable=0)Įprobit attack age bmi, select(full = age bmi i.checkup) entreat(exercise = bmi i.gym) vce(robust)Įstat teffects, atet subpop(if exercise=0)Įprobit attack age bmi, select(full = age bmi i.checkup) entreat(noexercise = bmi i.Propensity score matching is typically used to estimate the average treatment effect for the treated while inverse probability of treatment weighting aims at estimating the population average treatment effect. It’s no coincidence that this is the average of Alfred’s and Chizue’s treatment effects, ( 1 + 3) / 2 2.
Average treatment effect on the treated manual#
*Example from the Stata Manual on Extended Regression Models/ ERM (Example 4b, page 263) We can see in Table 10.3 that we get an average of ( 2 + 5) / 2 3.5 among the treated people, and ( 1 + 2) / 2 1.5 among the untreated people, giving us an effect of 3.5 1.5 2.

In a randomized trial, the average treatment effect can be estimated from a sample using a comparison in mean outcomes for treated and untreated units. The ATE measures the difference in mean outcomes between units assigned to the treatment and units assigned to the control. Addendum: I have tested a number of different examples by now, so I can say with a certain confidence that the easiest way to get the Average Treatment Effect on the Untreated (ATU) is to type:Įstat teffects, atet subpop(if treatmentvariable =0)Īs an alternative, using a non-treatment variable leads to the same results, but requires three additional steps:ī) running a new regression model with the generated variableĬ) switching the algebraic sign of the resulting ATET (sign of the margin, z-value and confidence interval).įor those who want to see for themselves, I have added a do-file with an example from the Stata Manual on Extended Regression Models (Example 4b, page 263) The average treatment effect is a measure used to compare treatments in randomized experiments, evaluation of policy interventions, and medical trials.
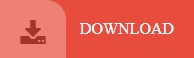